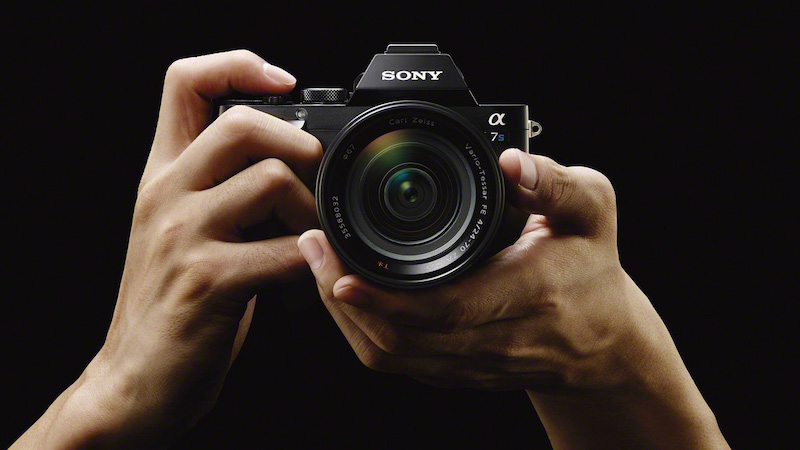
The Camera Uses AI To Take Color Photos In The Dark
Humans are not great at seeing in the dark. A paper describing the new camera, published in PLOS ONE journal, explains: “Some night vision systems use infrared light that is not perceptible to humans and the images rendered are transposed to a digital display presenting a monochromatic image in the visible spectrum.”
The paper went on to elaborate: “We sought to develop an imaging algorithm powered by optimized deep learning architectures whereby infrared spectral illumination of a scene could be used to predict a visible spectrum rendering of the scene as if it were perceived by a human with visible spectrum light.”
It went on: “This would make it possible to digitally render a visible spectrum scene to humans when they are otherwise in complete ‘darkness’ and only illuminated with infrared light.”
The algorithm is the important part here, as it’s what reconstructs the image using neural networks, a deep learning algorithm that is meant to replicate how the human brain learns. These algorithm were trained to see correlations between how the images look in infrared and also under the visible spectrum.
The paper said: “We … optimized a convolutional neural network with a U-Net-like architecture [an architecture designed to allow fast and precise image processing] to predict visible spectrum images from only near-infrared images. This study serves as a first step towards predicting human visible spectrum scenes from imperceptible near-infrared illumination.”
As of now, the scientists have admitted that it’s still just a “proof-of-principle-study using printed images with a limited optical pigment context.”
Professor Adrian Hilton, Director of the Centre of Vision, Speech, and Signal Processing (CVSSP) and University of Surrey said: “Human faces are, of course, a very constrained group of objects, if you like. It doesn’t immediately translate to coloring a general scene. As it stands at the moment, if you apply the method trained on faces to another scene, it probably wouldn’t work, it probably wouldn’t do anything sensible.”
He said in an interview with New Scientist: “I think this technology could be used for precise color evaluation if the amount and variety of data used to train the neural network is sufficiently large to increase accuracy.”
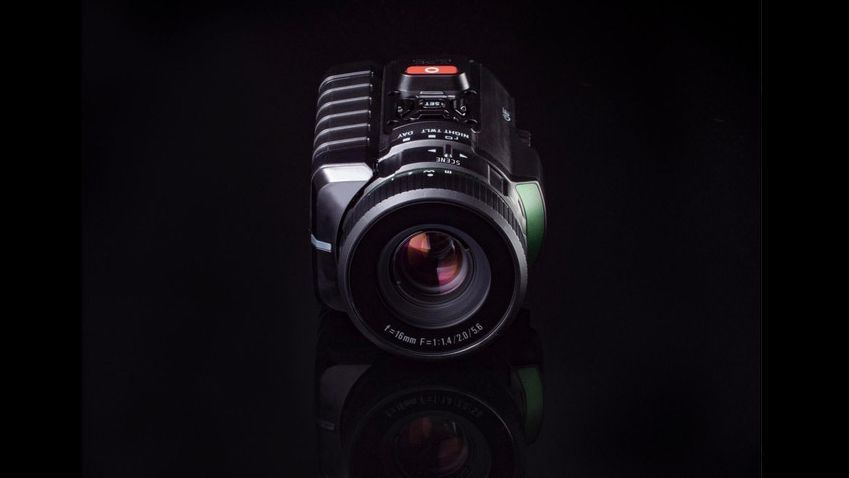
Color Is Recreated By The Algorithm