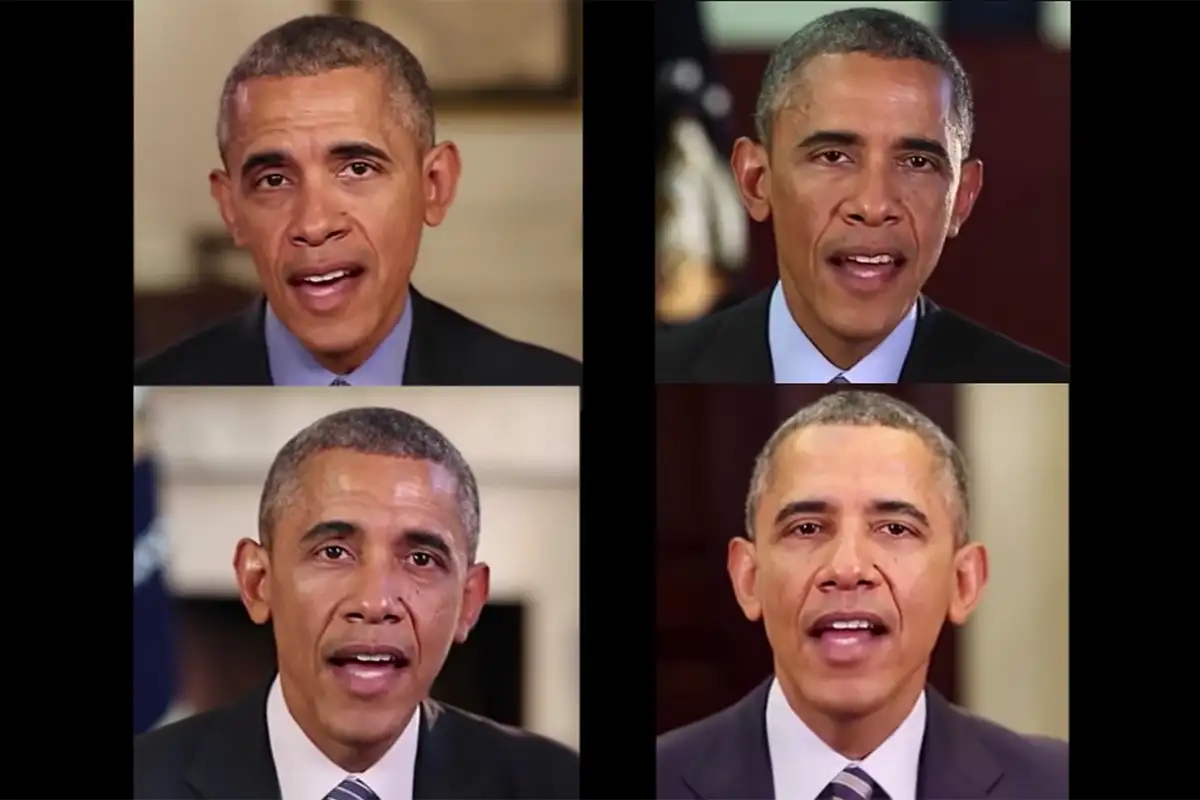
Deepfakes Are Becoming More Common
The process begins with the generator network crafting synthetic content, such as a face or voice, using a dataset of real examples as a reference. This generator then presents its creation to the discriminator, whose role is to distinguish between authentic and synthetic content. Through countless iterations, both networks refine their abilities, with the generator aiming to produce content that is increasingly indistinguishable from reality and the discriminator becoming more adept at telling the difference.
Training deepfake models often requires vast datasets of facial expressions, movements, and voice variations to capture the nuances of the targeted individual. As the algorithms learn from diverse samples, they gain the ability to replicate not only physical appearances but also subtle idiosyncrasies, making the resulting deepfake content remarkably realistic. This capacity to generate convincing simulations of real individuals has raised concerns about the potential for misuse, ranging from deceptive videos to identity theft.
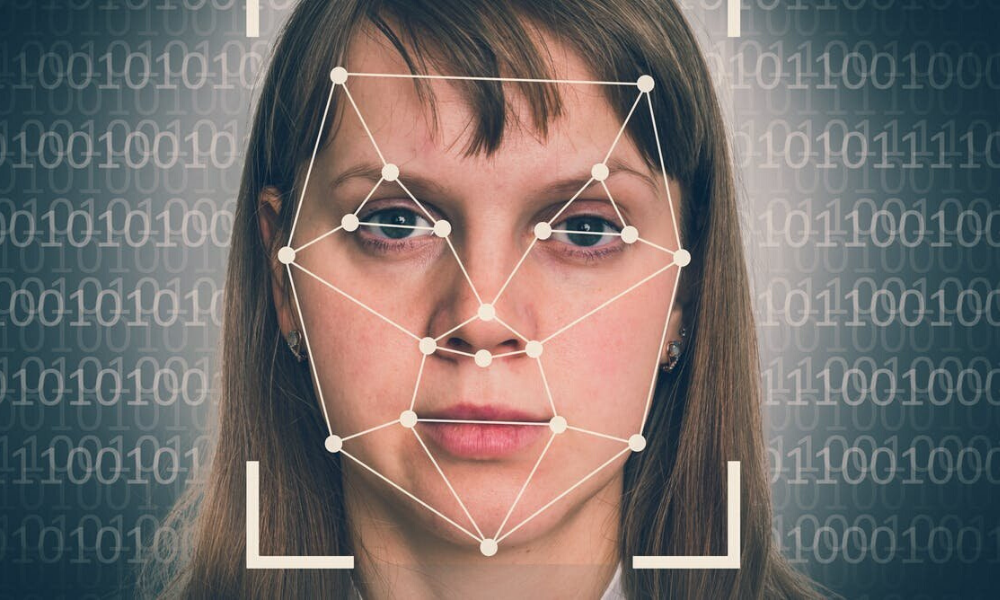
They Can Look Quite Real
The technology behind deepfakes doesn’t limit itself to visual content alone. Voice synthesis models, powered by recurrent neural networks and other advanced techniques, can mimic the cadence, tone, and even the unique vocal characteristics of a target individual. By analyzing extensive audio datasets, these models can produce eerily accurate imitations, further blurring the line between reality and manipulation.
While deepfake technology presents a myriad of ethical challenges, it also showcases the incredible potential of artificial intelligence. The responsible development and deployment of these technologies demand a delicate balance between innovation and ethical considerations. As researchers and policymakers grapple with the implications of deepfake technology, ongoing efforts are crucial to stay ahead of potential abuses and ensure that safeguards are in place to protect against malicious uses of this powerful AI-driven tool.
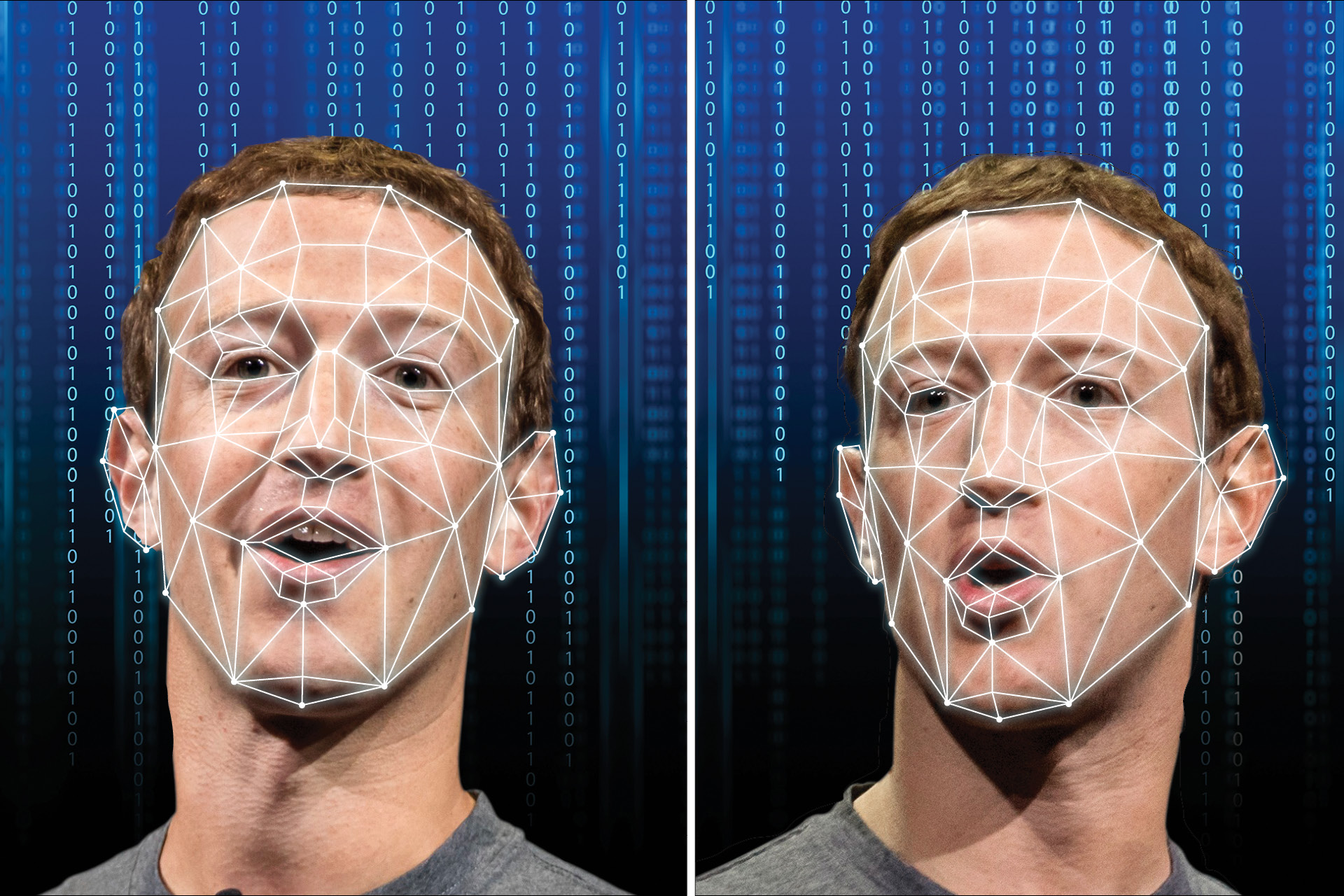
How To Tell If You’re Looking At A Deepfake